In the run-up to the 2023 IoTSF annual conference we invited Tim Snape, Artificial Intelligence Group and chair of the conference panel session: ‘Strategy, Ethics and Governance in the Age of AI-Powered Cybersecurity’, to give us some insights into the discussion in advance.
The following is the first part of a 2-part blog which acts as a primer to the discussion we’ll be having. Part 1 seeks to explain the challenges we face with AI, IoT cybersecurity and regulation and in part 2 we explore the solutions space.
It’s a highly nutritious read – Part 1: from traditional AI to bullshit generators and the formidable challenge of regulating AI
Grab a coffee and settle in!
Over to you Tim…
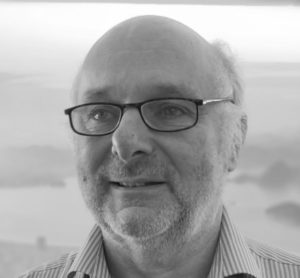
Tim Snape, Artificial Intelligence Group Ltd
Ever since my childhood, I’ve been hearing about the imminent arrival of Artificial Intelligence (AI) that will revolutionise the world when it finally does arrives.
My father, a lecturer of Mathematics and Physics at Manchester University, used to regale me with tales of his work on the computer’s being developed at Manchester in the early 1950’s .
One particular story stood out, involving a consulting project he undertook for the Lyons Company, who commissioned and funded the construction of the LEO computer. In this endeavour, he told me a story of how he used to program the LEO computer to perform matrix inversions, and what fascinated me most was the practical application of this process. He explained to me that by inverting a matrix of input variables, he could identify factors that could be used to predict future outcomes. This ability to foresee the future through a computer’s calculations seemed incredible to me, even though I didn’t fully grasp the mechanics at the time.
In hindsight, I now understand that he was employing a technique known as Linear Regression Analysis, a method that allows one to forecast future events based on input parameters and historical results data. This technique has been a cornerstone of predictive analytics for the past seven decades. By fine-tuning the algorithm to improve its accuracy with new data, it can become increasingly accurate in predicting future events. This method has remained central to predictive analytics. Today, we’re accustomed to training AI systems with datasets, enabling them to identify key variables in input data that influence specific outcomes. Once trained, we assign weightings to each input variable based on the training results. With these weightings, we can feed in new data and obtain a probability-based prediction of future events—a remarkable capability.
Even in the present day, I find this ability to predict events, like when a washing machine’s motor might fail or the likelihood of rain tomorrow, quite magical. Such predictions have become so commonplace that we hardly think twice about them now.
However, there’s a catch—the quality of predictions is only as good as the quality of the training data. If the training data is biased or insufficient, the predictions made using the model on real-world data will reflect these limitations.
Thankfully, data scientists have developed techniques to assess model accuracy. In the simplest scenario, multiple training sets with known outcomes are used. One set is used for training the predictive model, while the other sets are used to quantify its performance. Variations of this approach help quantify the completeness of a training set and its likely accuracy.
When referring to AI, I term this as “Traditional” or “Old-Fashioned” AI. It relies on well-understood statistical techniques for data analysis and reporting.
For many years, Traditional AI remained stagnant. It was a powerful set of tools but never truly achieved the level of intelligence that we humans understand as being “intelligence”. This changed dramatically at the beginning of this year, when OpenAI and the front-end tool ChatGPT, started to gain widespread attention.
I vividly remember my first experience using ChatGPT—I was astounded. Here was a system that appeared to comprehend my inquiries and could generate seemingly intelligent responses, even to obscure and esoteric questions. My amazement grew with every interaction.
However, it took me a while to realise that what I was encountering was essentially a “bullshit generator.” While it generated highly convincing human-like responses, it ultimately produced content lacking in accuracy.
The underlying mechanism used by ChatGPT, OpenAI, operates using a predictive text mechanism trained on an immense corpus of documents, allowing it to string together responses by predicting the most probable sequence of words. This process is supervised by a neural network with the objective of generating the desired response. And therein lies the issue—OpenAI is designed to provide responses that align with what the user wants to hear, which is not what most people expect or want. The consequence of this is, you cannot trust the accuracy of ChatGPT responses, you need to fact check the results from these queries.
The capabilities offered by Large Language Models are new and very different to those of the older Traditional AI technologies and it is important to understand this difference. What we have created with LLMs is the ability for machines to interact with humans and human data in a human like way. This human like capability is likely to result in new and emergent functions, some of which are likely to benefit mankind but there are concerns that technology will be misused, with some people believing it represents an existential threat to man kind.
This model is considered problematic for several reasons:
- Misinformation and Manipulation: The model’s ability to generate coherent and contextually relevant text makes it a potent tool for spreading misinformation and propaganda. Malicious actors can use it to create convincing fake news, misleading content, or deepfake text that can deceive individuals and manipulate public opinion.
- Amplification of Bias: Like many AI models, it is trained on vast amounts of data from the internet, which can contain inherent biases. This means that the model can inadvertently perpetuate and amplify these biases in its responses, leading to unfair or discriminatory outcomes in areas such as race, gender, and culture.
- Privacy Concerns: The model can generate text based on any input, and in some cases, it might inadvertently reveal sensitive or personal information if not properly controlled. This could lead to privacy breaches and the exposure of confidential data.
- Weaponisation: In the wrong hands, this model can be used for harmful purposes, including cyberattacks, phishing scams, and other cybercrimes. It can generate convincing messages to deceive individuals and gain unauthorised access to systems or personal information.
- Eroding Trust: As the model becomes more prevalent, it can erode trust in online communication. People may become skeptical of the authenticity of online content, leading to a general atmosphere of uncertainty and doubt.
- Undermining Expertise: The model’s ability to generate content on a wide range of topics might undermine the authority of experts and professionals in various fields. People may rely on AI-generated content without critically evaluating its accuracy or credibility.
- Social and Ethical Implications: The rapid development and deployment of AI models like this one often outpaces the establishment of appropriate regulations and ethical guidelines. This can lead to unintended consequences and challenges in addressing the societal impacts of such technology.
- Deepfakes and Manipulated Media: While this model primarily generates text, it can contribute to the creation of more sophisticated deepfake content when combined with other AI technologies. This includes deepfake videos and audio recordings, which can further deceive and manipulate individuals.
- Loss of Jobs: In some industries, the use of AI-generated content might lead to job displacement, as automated systems can produce content more efficiently and inexpensively than human workers.
- Dependency on AI: Over reliance on AI models for decision-making and information retrieval can lead to a reduction in critical thinking and research skills among individuals, potentially hindering their ability to evaluate information critically.
Implementing effective legislation to address these concerns and fears, so that it does not stifle innovation is a complex and multi-faceted challenge. Governments have been struggling with this problem for some time, trying to resolve the gap between what is desirable and what is viable.
Key challenges and considerations that need to be highlighted :
- Rapid Technological Advancements: AI technologies are advancing rapidly, and legislation can struggle to keep pace. By the time a law is enacted, it may already be outdated due to emerging AI developments. This necessitates a flexible legislative framework that can adapt to evolving AI capabilities.
- Balancing Innovation and Regulation: Striking the right balance between fostering innovation and regulating AI is challenging. Overly strict regulations can stifle AI development, while lax regulations can lead to risks and abuses. Achieving this balance is crucial for maintaining competitiveness while ensuring safety and ethical use of AI.
- Global Coordination: AI operates globally, making it difficult for individual countries to regulate effectively. Achieving international coordination and harmonisation of AI regulations is essential to avoid conflicts and ensure consistent standards across borders.
- Resource Allocation: Enforcing AI regulations requires resources, including funding, skilled personnel, and technology. Governments and regulatory bodies must allocate resources efficiently to monitor and enforce compliance, which can be costly.
- Ethical Considerations: AI legislation often includes ethical principles that require careful consideration. Balancing ethical concerns with practicality and feasibility is challenging. Moreover, ethical principles can vary across cultures, making it challenging to create universally applicable regulations.
- Accountability and Liability: Determining liability in cases of AI-related harm can be complex. Legislation must establish clear rules for attributing responsibility when AI systems malfunction or cause harm, which may involve multiple parties, including developers, users, and organisations.
- Access to AI Expertise: Developing and implementing AI regulations requires expertise in both technology and policy. Governments and regulatory bodies must ensure they have access to AI experts who can guide the legislative process effectively.
- Adaptability to Emerging Risks: Legislation should be future-proofed to address unforeseen risks associated with AI, including those that may emerge as AI technologies evolve. This requires ongoing monitoring and revision of regulations.
- Public Engagement: Effective AI legislation should involve input from a wide range of stakeholders, including industry experts, civil society, and the general public. Public engagement can be resource-intensive but is crucial for creating regulations that reflect societal values and concerns.
- Enforcement and Compliance: Developing regulations is only the first step; ensuring compliance and enforcement is equally important. Legislation that is not enforced or not enforceable has little value, indeed it may be counterproductive.
From a Cyber and Compliance perspective, there are two dimensions to the issue – the good and the bad.
Potentially AI creates the opportunity to create software that is more reliable and robust, and more resilient and resistant to Cyber threats. There have been numerous statistics claiming productivity benefits for developers using AI to support code creation. My own experience has been that providing I can express and describe the code I want produced, my AI generated code is of far higher quality than my human generated code – and it generates the code I would take three days to generate – in seconds.
Not only does it generate good quality code, but it can also generate test data and documentation for that code.
Given a new exploit, one can envisage AI tools being used to scan multi-million lines of code to locate and identify exploitable code, weaknesses and defects. Augmenting Static code analysis tools, integrating them with AIs will make them far more capable at identifying code that does not meet secure by design requirements.
It does appear that we are about to enter a new world where white hat programmers will not write code anymore, instead they will describe the code they require and the AIs will create it for them.
But what of the Black Hat programmers? AI will empower the bad guys to discover vulnerabilities in source and binary code and create ever more sophisticated exploits of them. Whereas before a script kiddy would use a buffer overflow attack to crash a system, the AI empowered kiddies, will now be able to engineer data into the overflowing buffers to perform really nasty and insidious exploits. The only defence will be to ensure that the software that you produce is hardened such that it is resilient to AI supported attacks.
In short we are entering an arms race, where the legacy code produced by humans over the last few decades is going to be vulnerable to attack and there will need to be a major drive to review and update legacy code bases.
The answer that is being proposed by Governments is Legislation. The EU AI Act is focused on AI but in parallel we have existing Privacy legislation and new requirements to ensure that software used in Critical Systems is free of vulnerabilities. If you view the requirements and the solutions not just in terms of AI requirements, but you also add the GDPR and the Cyber Resilience Act requirements as well, what you find is that AI has created the situation where all these areas of Compliance merge and overlap. And organisations that get it wrong will face eye watering penalties.
In the world of IOT there is an additional concern. A large percentage of IOT systems cannot be patched. They are remote and isolated running on highly constrained hardware. Implementing the code functionality to support the patching requirement, is not easy. In many cases, if you are running on really low end hardware, it is simply not possible to create a solution that can be remotely patched. If you must patch these systems, then the only answer is to return them to the vendor and for an engineer to reload an updated code image.
It’s difficult to imagine what is going to happen, IOT services that cannot be patched are in breach of requirement three, in the ETSI EN 303 645 standard – To Keep Software Updated. If you then factor in requirement two, in the same standard. The Requirement to report Vulnerabilities – we have created the self defeating situation, where IOT suppliers will be required to report exploitable vulnerabilities for which there is no remedy.
In conclusion, the development and implementation of effective AI legislation that balances innovation with safety and ethics is a formidable challenge. It requires a nuanced approach that considers the rapidly evolving technological landscape, global coordination, resource allocation, ethical considerations, and the ability to adapt to emerging risks. Achieving these goals while maintaining cost-effectiveness is an ongoing endeavour that necessitates collaboration between governments, industry, and civil society to ensure AI benefits society while mitigating its risks.
In the next article – Transparency by Design: Making AI Work for the Benefit of Society – I try to define what will be needed in order for regulations to be both enforceable and practical such that they address the concerns that are being raised and explore how this maximises the benefits that AI potentially offers.
The IoTSF Conference takes place on November 7th at the IET in London with the theme: Securing the Internet of Things in the Era of Artificial Intelligence – come along, you’re invited!